Short bio: Ana Luisa Papoila holds a degree in Mathematics from the Faculty of Sciences at the University of Lisbon (FCUL), a master's degree in Statistics and Optimization from the Faculty of Sciences and Technology at the Nova University of Lisbon (FCT-UNL), and a PhD in Probability and Statistics from FCUL. She is a Full Professor at NOVA Medical School/Faculty of Medical Sciences at UNL, and a member of both the Scientific Council and the Faculty Council of this institution. She is an integrated member of the Center of Statistics and its Applications at the University of Lisbon (CEAUL), having also been part of its Executive Committee. She is a member of the Portuguese Society of Statistics and a member of the General Assembly Board of this Society. Her main area of interest lies in statistical methodologies applied to Health Sciences, and she has also contributed to enhancing the performance of generalized additive neural networks and improving the interpretability of their results. She is part of the team that led the creation and organization of a research center at the former Centro Hospitalar Universitário de Lisboa Central, which includes six hospitals. She has been involved in medical research projects that resulted in several articles published in international journals and awards, including the 1996 Bial Award for Clinical Medicine.
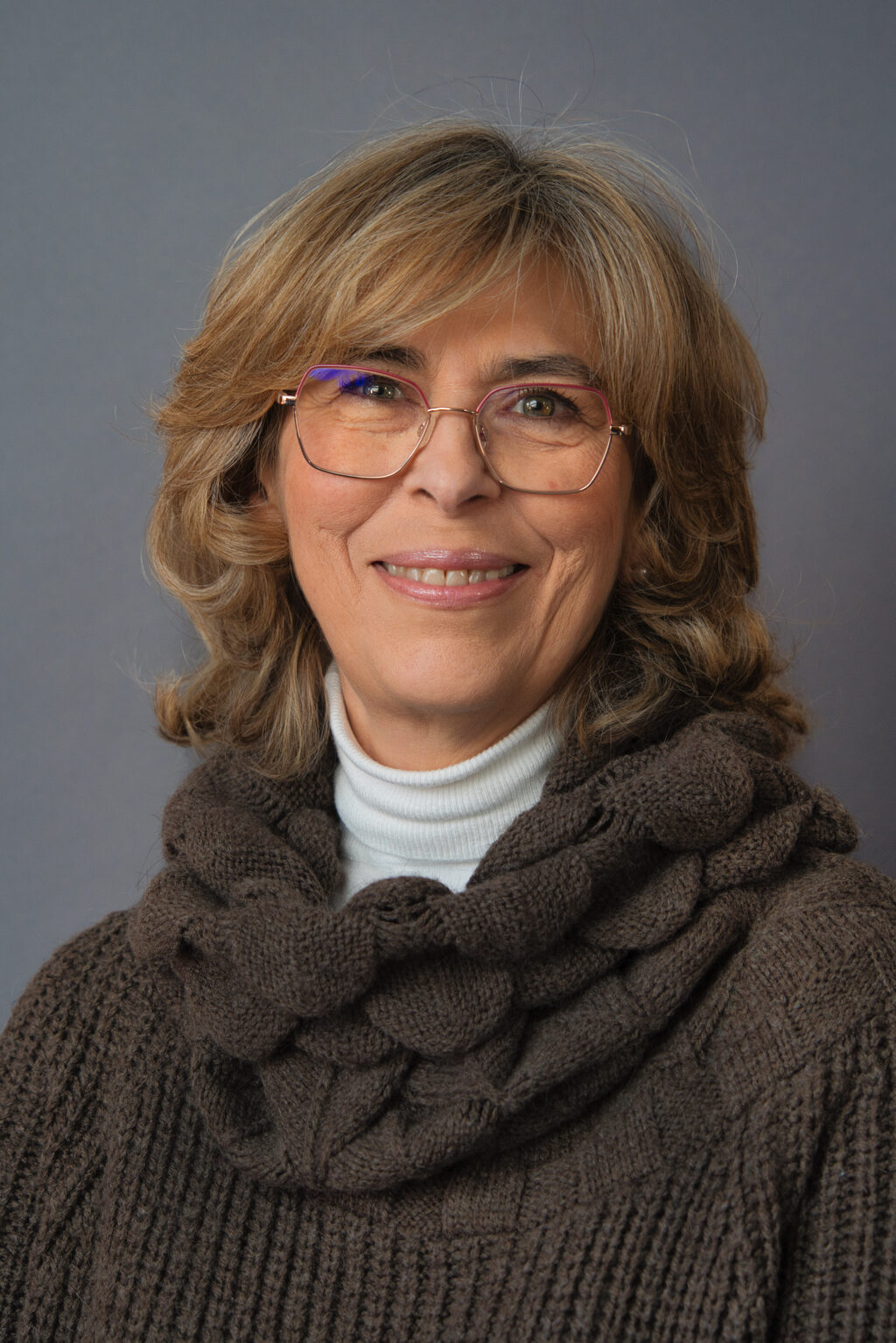
Statistics and Artificial Intelligence in Medicine
The growing role of artificial intelligence (AI) in medicine is extremely promising, though it comes with its own challenges. Among many others, I emphasize the lack of transparency in AI and those more problematic issues related to clinical practice.
Despite impressive results in areas like disease diagnosis, image processing, and other medical applications, AI systems still struggle to replicate the depth of human knowledge, expertise and clinical sensitivity, necessary for better decision-making. To address the transparency issue, many researchers have turned their attention to Explainable AI (XAI) techniques, which aim to clarify both the algorithms and the results produced by AI systems. This talk will focus on the crucial role of statistics in the development of AI models and examine how machine learning tools, such as artificial neural networks, can improve both performance and interpretability through the integration of statistical methods.
Within the general aim of extreme value statistics lies the estimation of an event that is so rare that might have never been witnessed in the past. While parametric estimation of an extreme quantile has now climbed to the lore of risk assessment, especially in terms of return levels by way of hazard curves, analogous non-parametric statistical methodology is far less explored. This creates an interesting topic, in part because there are distinct albeit equivalent ways to define an (extreme) out-of-sample quantile supported by different constructs rooted in the same foundational extreme value theorem.
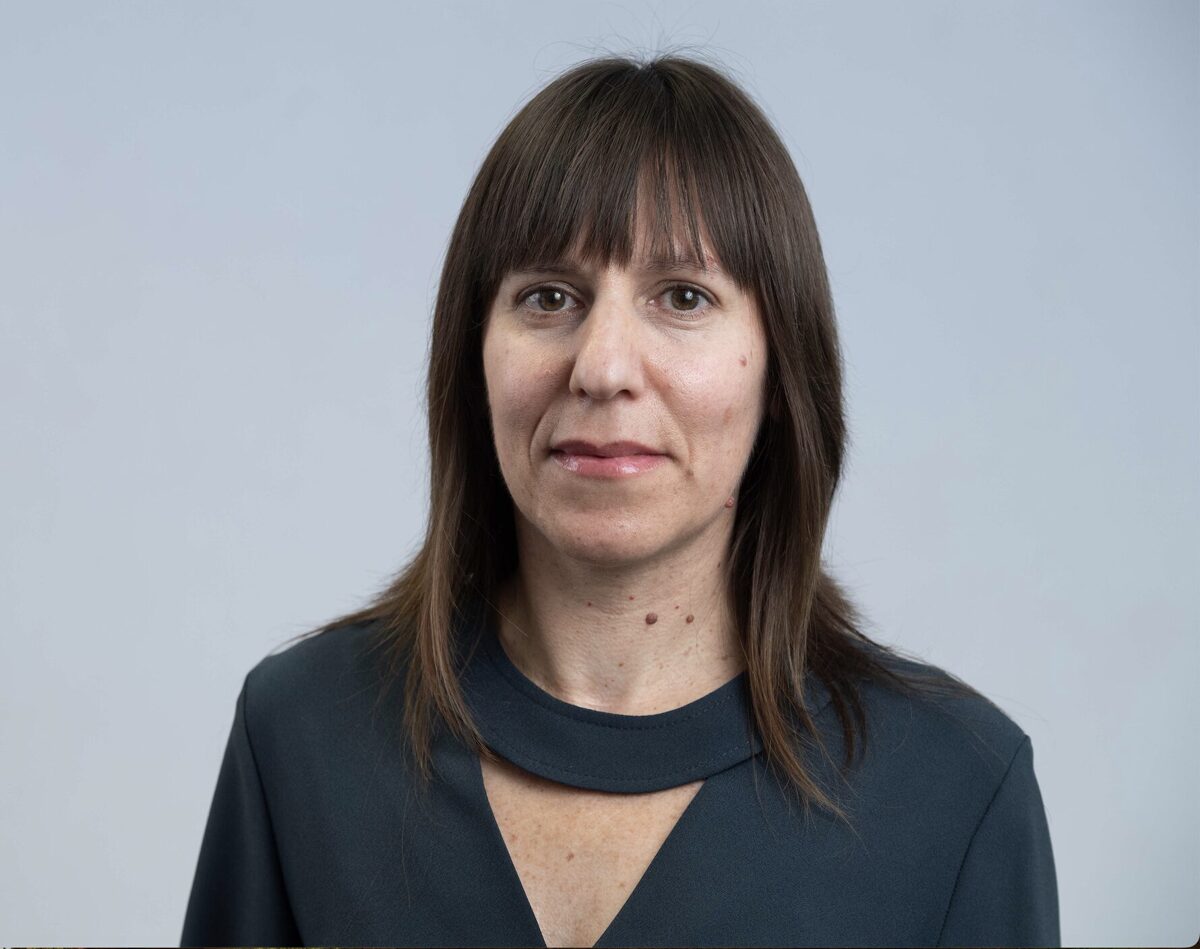
Short bio: Claudia Neves joined the Department of Mathematics at King’s College London as Lecturer in Statistics in 2021 where she has stayed since rising to Senior Lecturer in 2023. Prior to this, she was a Lecturer in Theoretical Probability and Statistics at the University of Reading during which time she held an EPSRC Innovation Fellowship for the project "Multivariate Max-stable Processes with Application to the Forecasting of Multiple Hazards". Before joining Reading, she was Assistant Professor at the University of Aveiro, Portugal. Her primary field of expertise is in extreme value theory for modelling extreme and rare events. Her research centres around the development of statistical methodology to help solve challenging problems in several fields of applied sciences, ranging from meteorology and the environment to insurance and finance. Claudia earned her PhD in Statistics and Operational Research in 2006 from the Faculty of Science, University of Lisbon. As well as holding a Visiting Research Fellowship at the University of Reading, Claudia has maintained to date strong links with academics and researchers based in Lisbon through her continued collaboration with the Centre of Statistics and its Applications. Since 2024, Claudia is Visiting Associate Professor in the Department of Statistics and Operational Research at the Faculty of Science of the University of Lisbon.
Explanatory Model Analysis
During the talk, I will present the recent development of techniques for visualization and exploration of predictive models, developed under the terms interpretable machine learning (IML) / explainable artificial intelligence (XAI). I will present the most popular approaches used for model exploration (attribution methods, variable profiles, variable importance, Rashomon set analysis) and demonstrate their usefulness using real medical data analysis as an example. I will conclude the lecture with a brief discussion of current challenges and open problems that we need to solve along the way to Responsible ML.
Short bio: Przemyslaw Biecek (PhD in Mathematics, professor in Computer Science) is the leader of the MI2.AI research group, which conducts research in the area of explainable artificial intelligence. Over the years, he has conducted research related to visualization and exploration of data, and today, he uses these experiences to create new approaches to visualizing, exploring, and explaining predictive models. Before academia, he worked as a principal data scientist at Samsung and led the Data Visualization Group at Netezza. His main achievements are summarised in the Explanatory Model Analysis (https://ema.drwhy.ai/) monography and open package DALEX for R and Python (https://dalex.drwhy.ai/), which provides descriptive and model-agnostic local explanations for machine learning models. Trivia: in his spare time, he creates comics about data exploration and visualization, such as Charts Runners (https://betaandbit.github.io/Charts/) or The Hitchhiker’s Guide to Responsible Machine Learning (https://rml.mi2.ai/) and many others.
The unreasonable effectiveness of data science
There are three factors responsible for the revolution brought about by artificial intelligence: (1) the constant increase in computational capacity; (2) the accumulation of large amounts of data generating insights and enabling the creation of data-driven products; (3) the development of statistical learning theory and its algorithms. The alignment of these planets allowed great success in difficult tasks such as the development of virtual assistants and chatbots, self-driving car, the automatic translation between languages, and the early detection of unspecified anomalies in vital signs. In this talk, I will present an overview of these developments from a historical point of view focusing on the contribution brought by Statistics. I will illustrate this presentation with examples from my own research on epidemiological surveillance using social media data, space-time demographic forecasting, and the Bayesian spatial partitioning of space-time maps.
Short bio: Renato Assunção received his Ph.D. in Statistics from the University of Washington in 1994. He is a researcher at ESRI Inc., Redlands, USA since 2021, and a full professor in the Computer Science Department, at Universidade Federal de Minas Gerais (UFMG), Belo Horizonte, Brazil, where he is affiliated since 1988. His research focuses mainly on the development of algorithms and statistical methods for the analysis of spatial data, specially areal and point processes data. It is primarily concerned with the spatial analysis of risk appearing in many fields such as epidemiological surveillance, sensor networks, and demographic problems.